

A 3D face, described by points in x, y and z undergo a transformation and the demixing process recovers the transform and the original shapeĪ solution to the noise-free SfM problem in (3), on which Tomasi and Kanade's original factorization technique ( Tomasi and Kanade 1992) is based, is provided from the Karhunen-Loeve expansion-the eigenvectors of Σ w uniquely diagonalize it, and provide an orthonormal basis for w. Structure from motion as a demixing problem. Similarly we will use the terms mixing matrix and motion matrix interchangeably. Note that we will use the terms sources and shape interchangeably when describing blind source separation as it is applied to SfM. This is functionally identical to the process of recovering three 1D signals (sources) which were assumed to be mixed by a process which generates a set of 1D mixtures.
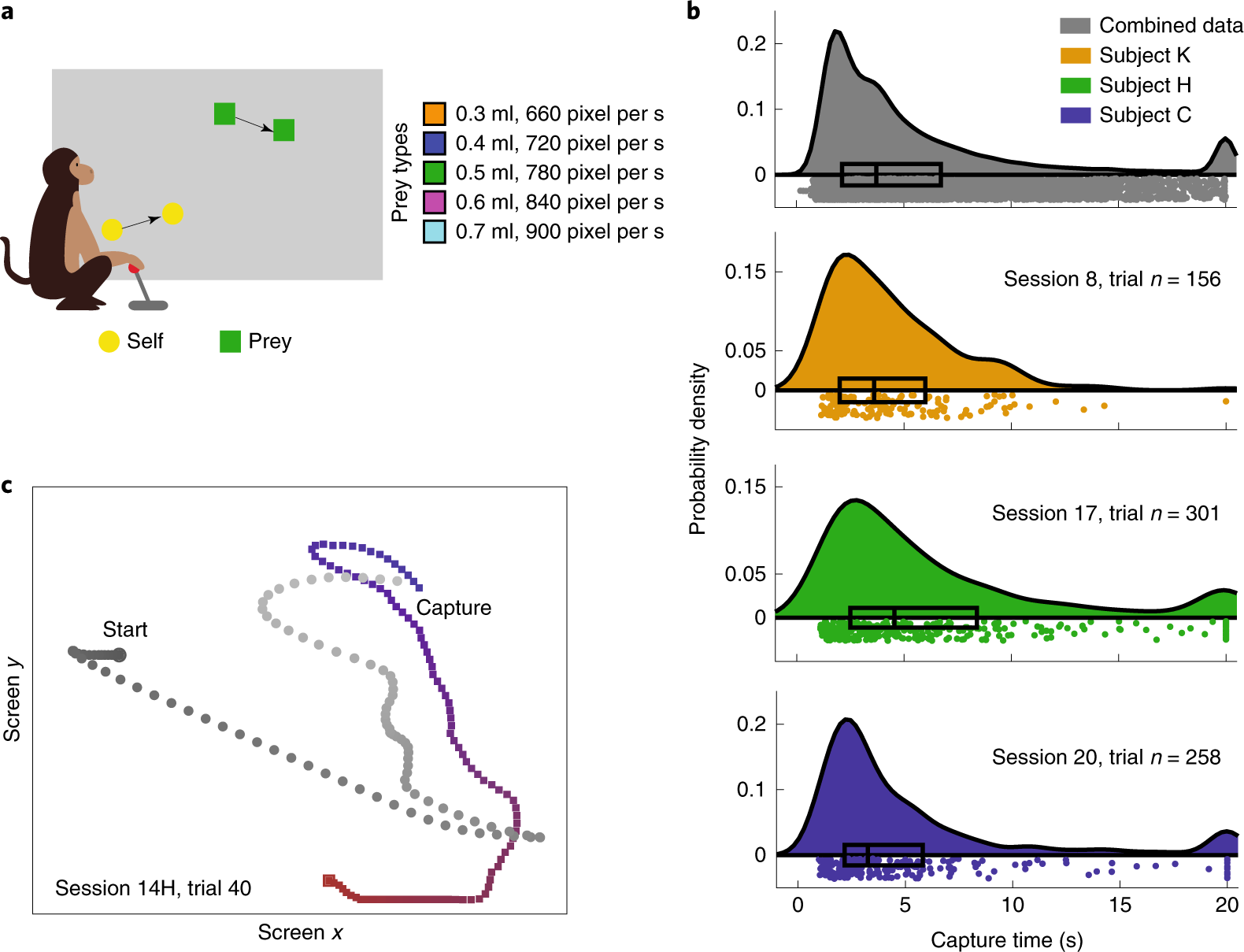
SfM seeks to undo the generative process by recovering the transformation (demixing matrix) and the 3D shape, described by recovered 1D measurements in x, y and z, that generated the set of 1D observations. This describes the generative process of the image sequence of observed points for an object under motion. Again, the 2D observations can be described as a sequence of 1D point observations for x and y measured from the image sequence. In SfM, these points are assumed to undergo a transformation which includes the description of the motion and the 3D to 2D transform which generates a set of 2D observations for each frame (2D mixtures) of an image sequence of the motion. As the figure shows, when a 3D object is described by a set of 3D points on the object's surface, these points can be described by an unordered sequence of 1D measurements for the x, y and z directions. The relationship between the sources and observations of a general blind source separation problem and the factorization of shape and motion in structure from motion in (3) is shown in Fig. To determine R and s in the noise-free case we seek a solution where the covariance matrix of w, denoted Σ w, is rank 3. The R matrix creates a linear mixture of s yielding the observations (mixture) w. Herein, we will focus on the use of higher-order statistics as a means of unmixing the sources and use the terms “ICA” and “blind source separation” interchangeably.Įxamining the stochastic description of SfM in (3), we see that it, too, can be described as a linear mixing problem. 1997 for an example that uses second-order statistics only). When the higher-order statistical properties of the sources s are used, the approach is generally called ICA, although there are a number of ways to perform blind source separation (see Belouchrani et al. The goal of blind source separation is to “unmix” s to recover the original 3D point without any knowledge of the distributions of R or s. In the signal processing community, blind source separation problems where a set of sources s are linearly mixed by a mixing process R are defined by the same model in (3), where w is a vector of observations of the mixtures of s. Where w is now a 2 F-dimensional random vector of (centered) observed points and R ∈ ℝ 2 F×3 is a transformation matrix over all frames, s is a three-dimensional random vector as defined above. We provide extensive experimental results on synthetic and real tracked points in order to quantify the improvement obtained from this technique.
#FMINUNC MATLAB 2009 PDF#
We demonstrate that it suffices to know whether the pdf is sub-or super-Gaussian (i.e., semi-parametric estimation) and derive a simple formulation to determine this from the data. A key result is that the blind source separation approach has the ability to recover the motion and shape matrices without the need to explicitly know the motion or shape pdf. A further advantage of viewing SfM as a blind source problem is that it easily allows for the inclusion of noise and shape models, resulting in Maximum Likelihood (ML) or Maximum a Posteriori (MAP) shape and motion estimates. Previous techniques have either explicitly or implicitly used only second-order statistics in models of shape or noise. Given that for almost all practical joint densities of shape points, the marginal densities are non-Gaussian, we show how higher-order statistics can be used to provide improvements in shape estimates over the methods of factorization via Singular Value Decomposition (SVD), bundle adjustment and Bayesian approaches.
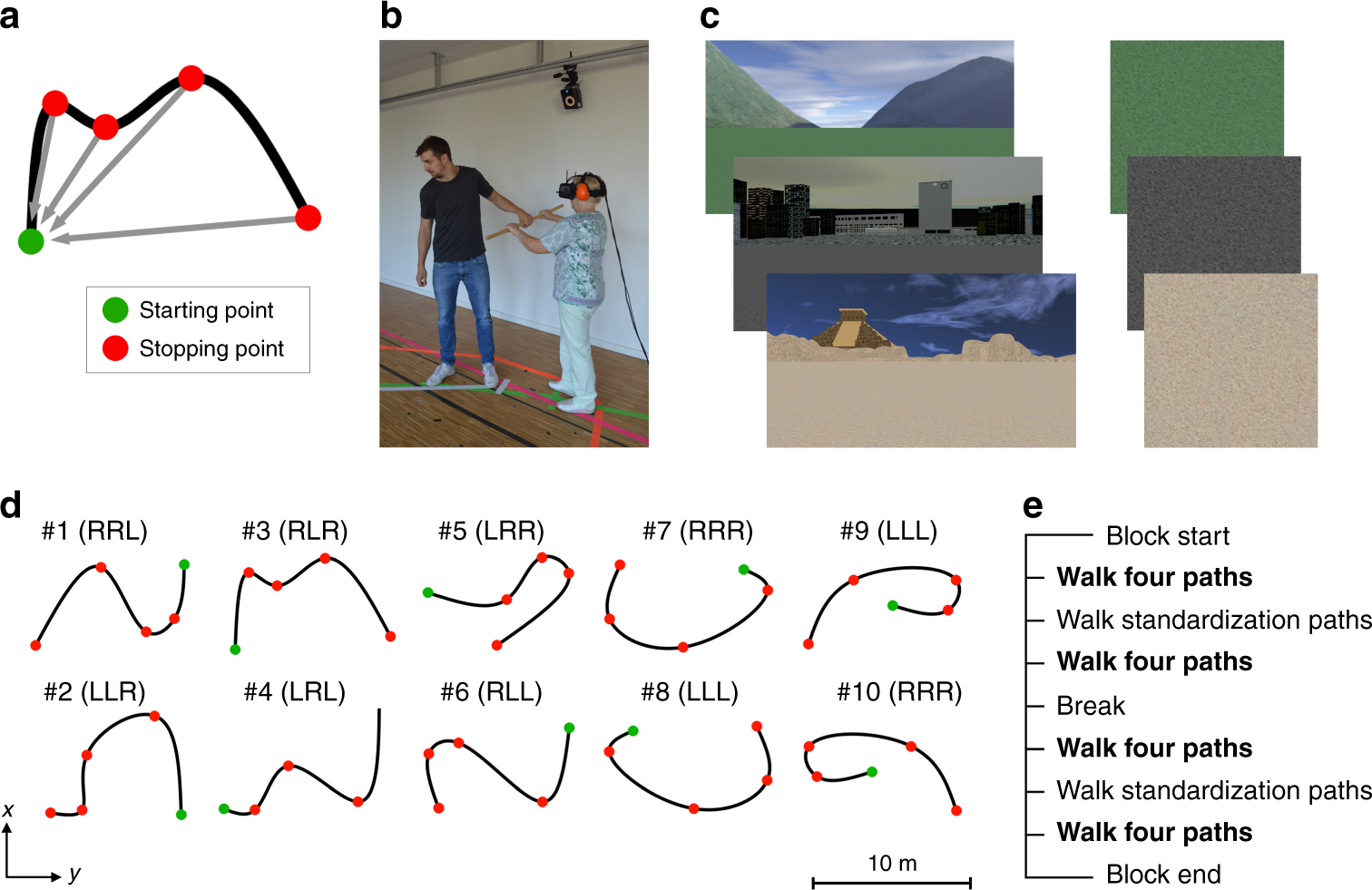
We present an information theoretic approach to define the problem of structure from motion (SfM) as a blind source separation one.
